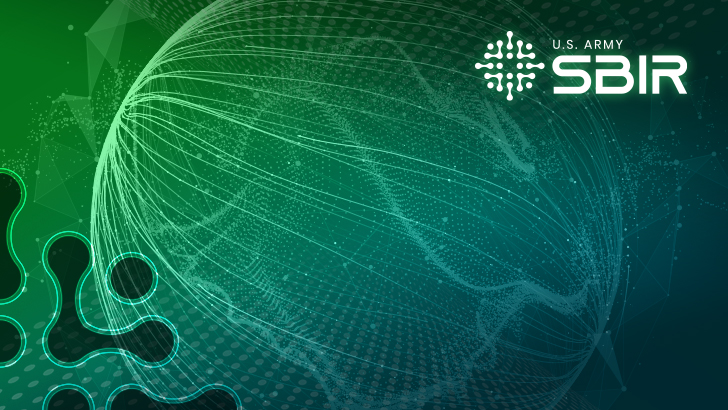
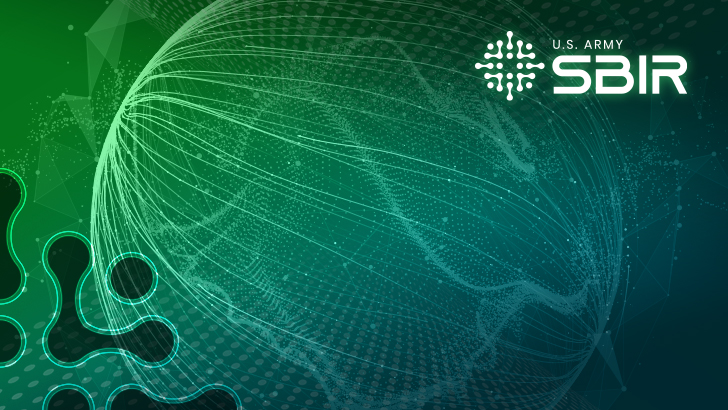
Objective
US Army Pacific executes Operation Pathways within the INDOPACOM AOR and the multitude of languages, populations, and cultures within the INDOPACOM AOR. To provide effects that instantiate and reinforce the INDOPACOM Desired Perceptions (available at the SECRET//NOFORN Level), the Theater Army requires a range of Natural Language Processing (NLP) capabilities from Machine Translation to stance detection and summarization technologies to both produce effects and assess the information environment for the range of Low Resource Languages resident within this AOR.
Description
Recent relevant research on this topic focuses on languages within Western, educated, industrialized, rich, and democratic demographic populations. But due to the emergence of Large Language Models such as GPT-3 and 4 and BLOOM, generational improvements in Low Resource Language NLP capabilities are technically viable. USARPAC seeks to leverage those advances for languages resident in this AOR.
Most commercial access translation technologies through API and do not perform bespoke model training. Further, most commercial services perform higher level analysis (stance detection) on already translated media where best practices would necessitate development in the source language.
Computing resources are inexpensive and scalable and available training data is likely acquirable through crowd-sourced manual labeling. Some zero-shot approaches may be effective for lower-fidelity requirements. Further, this technology leverages LLMs available through open-source repositories and emerging techniques such as Dictionary-based Phrase-level Prompting of Large Language Models for Machine Translation.
By translating and assessing media in source languages, these technologies would enable reach into previously inaccessible populations and enable at-scale assessment tools rather than endure knowledge losses due to default to-English approaches.
Phase I
A successful Phase I will have a justifiable and solidified proof of concept for low resource language.
Phase II
Expected deliverables of this phase include a Deployed Machine Translation model. Testing and Evaluation would be executed in accordance with standard-practice metrics based on widely accepted and emerging evaluation benchmarks (FLORES) via GEMBA, Word Error Rate, Bilingual Evaluation Understudy, and other academic-grade metrics.
Phase III
Initial model development will transition to continuous training and development for use-cases specific to the transition partner, US Army Pacific. There is high dual-use potential for machine translation. The technology can be used by many industries as globalization occurs and multi-lingual communications become a priority.
Submission Info
For more information, and to submit your full proposal package, visit the DSIP Portal.
References:
Costa-jussà, Marta R., et al. “No language left behind: Scaling human-centered machine translation.” ArXivpreprint arXiv:2207.04672 (2022).
Hendy, Amr, et al. “How good are gpt models at machine translation? a comprehensive evaluation.” arXivpreprint arXiv:2302.09210 (2023).
Ghazvininejad, Marjan, Hila Gonen, and Luke Zettlemoyer. “Dictionary-based Phrase-level Prompting of Large Language Models for Machine Translation.” arXiv preprint arXiv:2302.07856 (2023).
Objective
US Army Pacific executes Operation Pathways within the INDOPACOM AOR and the multitude of languages, populations, and cultures within the INDOPACOM AOR. To provide effects that instantiate and reinforce the INDOPACOM Desired Perceptions (available at the SECRET//NOFORN Level), the Theater Army requires a range of Natural Language Processing (NLP) capabilities from Machine Translation to stance detection and summarization technologies to both produce effects and assess the information environment for the range of Low Resource Languages resident within this AOR.
Description
Recent relevant research on this topic focuses on languages within Western, educated, industrialized, rich, and democratic demographic populations. But due to the emergence of Large Language Models such as GPT-3 and 4 and BLOOM, generational improvements in Low Resource Language NLP capabilities are technically viable. USARPAC seeks to leverage those advances for languages resident in this AOR.
Most commercial access translation technologies through API and do not perform bespoke model training. Further, most commercial services perform higher level analysis (stance detection) on already translated media where best practices would necessitate development in the source language.
Computing resources are inexpensive and scalable and available training data is likely acquirable through crowd-sourced manual labeling. Some zero-shot approaches may be effective for lower-fidelity requirements. Further, this technology leverages LLMs available through open-source repositories and emerging techniques such as Dictionary-based Phrase-level Prompting of Large Language Models for Machine Translation.
By translating and assessing media in source languages, these technologies would enable reach into previously inaccessible populations and enable at-scale assessment tools rather than endure knowledge losses due to default to-English approaches.
Phase I
A successful Phase I will have a justifiable and solidified proof of concept for low resource language.
Phase II
Expected deliverables of this phase include a Deployed Machine Translation model. Testing and Evaluation would be executed in accordance with standard-practice metrics based on widely accepted and emerging evaluation benchmarks (FLORES) via GEMBA, Word Error Rate, Bilingual Evaluation Understudy, and other academic-grade metrics.
Phase III
Initial model development will transition to continuous training and development for use-cases specific to the transition partner, US Army Pacific. There is high dual-use potential for machine translation. The technology can be used by many industries as globalization occurs and multi-lingual communications become a priority.
Submission Info
For more information, and to submit your full proposal package, visit the DSIP Portal.
References:
Costa-jussà, Marta R., et al. “No language left behind: Scaling human-centered machine translation.” ArXivpreprint arXiv:2207.04672 (2022).
Hendy, Amr, et al. “How good are gpt models at machine translation? a comprehensive evaluation.” arXivpreprint arXiv:2302.09210 (2023).
Ghazvininejad, Marjan, Hila Gonen, and Luke Zettlemoyer. “Dictionary-based Phrase-level Prompting of Large Language Models for Machine Translation.” arXiv preprint arXiv:2302.07856 (2023).